Unlocking the Power of Synthetic Data for Large Language Models
- miza42
- May 16
- 2 min read
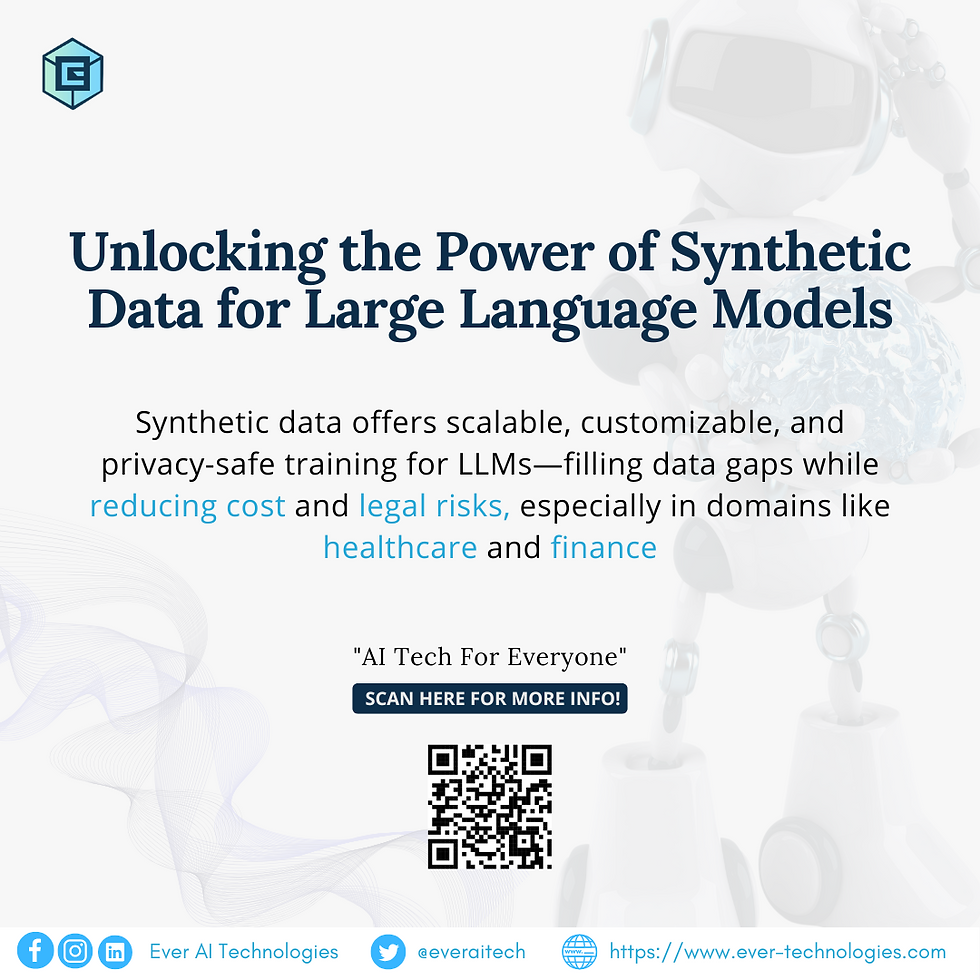
The need for high-quality training data has never been higher as large language models (LLMs) continue to change the AI landscape. There are a number of drawbacks to traditional data sources, such as books, websites, and user-generated information, such as copyright difficulties, data sparsity in specialized fields, and ethical dilemmas around data provenance. This is where synthetic data comes in: datasets that are created intentionally to resemble real-world examples. With its ability to improve or even replace traditional datasets, this new method is quickly gaining popularity.
Synthetic data's versatility is one of its main benefits. Synthetic data may be carefully adapted to certain activities, domains, or language styles, in contrast to fundamentally noisy and imbalanced real data. Do you want to train a medical conversation model without jeopardizing patient privacy? Conversations between the doctor and the patient can be artificially simulated. Do you need to develop a chatbot that can comprehend technical jargon or uncommon languages? When there are few real-world samples available, synthetic data might be used to fill in the gaps.
In addition to customisation, synthetic data provides a scalable and affordable training option. The time and cost associated with data collection and annotation can be significantly decreased by creating synthetic samples utilizing rule-based systems, simulations, or even simpler foundation models. Additionally, there are no legal limitations on updating or expanding synthetic datasets, which allows for a more flexible response to changing model needs.
Synthetic data is not a cure-all, though. The performance of the model might be harmed by biases, hallucinations, or artificial patterns introduced by poorly produced data. Combining strong quality controls and validation procedures with synthetic data is essential. The best of both worlds is frequently provided by hybrid training methods that combine synthetic and real-world data, striking a balance between diversity, scalability, and realism.
Synthetic data will become more and more important as the discipline develops in order to create language models that are performant, ethical, and domain-specific. Even while there are still obstacles to overcome, the capacity to customize data to suit particular requirements presents LLMs with fascinating new opportunities in a variety of sectors, including healthcare, finance, and education. In the future of AI, synthetic data is a strategic advantage for both academics and developers, not merely a fallback choice.
🚨 MAY PROMOTION🚨
Hey fam, we've got GREAT news just for you! 🎉
Get a MASSIVE 60% OFF – now only RM288 🎁
It’s our special Raya Promo, and it’s too good to miss! 🌙✨
📅 Promo valid until30th May –
Grab it while it lasts... Secure your seats now! 💨
Let’s level up together. Don’t say we didn’t tell you! 😉🔥

Comments